Several factors determine the evolutionary success of SARS-CoV-2 variants of concern. A recent publication looks into the factors influencing this success – the number of people that an individual with the virus infects in turn, the timing of these infections and the contact rate –, using mathematical modeling to help understand the characteristics of these variants.
Mutations in the SARS-CoV-2 virus have led to the emergence of variants of concern, like Alpha, Delta, and more recently Omicron. These strains contain genetic modifications that help the virus spread more easily and can alter the severity of the illness it causes. The variants have led to rising numbers of infections, known as epidemic waves, in many parts of the world.
Tracking new variants of concern is crucial to anticipating epidemic dynamics. To do this, scientists estimate how many people one person with the virus can infect, also known as the number of secondary infections. They may also measure when in the course of the infection an individual may pass the virus onto others. Together, these metrics help determine the speed and scale of an outbreak caused by a new variant. The more people the new variant infects and the more quickly it spreads, the more likely it is to replace existing viral strains.
So far, most studies have assumed that the growth rate of a new variant depends solely on the number of secondary infections that it causes, and the timing of secondary infections is often not considered. To address this, the scientists built a mathematical model that combines these two parameters to determine the growth rate of new viral strains.
The model showed that variants which rapidly cause secondary infections have a greater growth advantage over existing strains when the virus is more easily transmitted between individuals and the epidemic spreads rapidly. But when there is less transmission and the epidemic is declining, variants that generate secondary infections after a longer time have an advantage. For example, when control measures like mask wearing or social distancing are in place, a variant that causes delayed secondary infections may be more advantageous.
The scientists applied their model to data from the Alpha and Delta variant outbreaks in the United Kingdom. They found that Alpha and Delta did not change the timing of secondary infections compared to previously circulating strains. But the Alpha variant had a 54% transmission advantage over previous strains and the Delta variant had a 140% transmission advantage over Alpha.
These findings suggest that the timing of secondary infections and transmission rates both play an important role in how quickly a virus spreads. The new mathematical model may help epidemiologists better predict the trajectory of new SARS-CoV-2 variants and determine how to best control their spread.
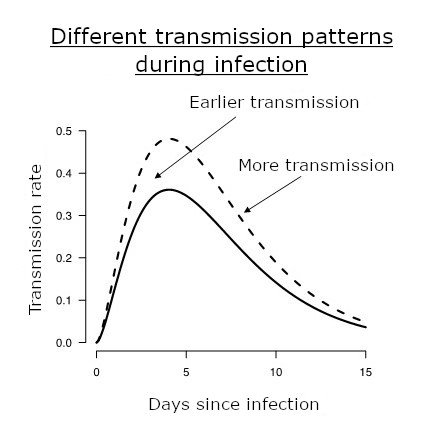
Different infectivity profiles are illustrated. The infectivity profile is the transmission rate of an infected individual as a function of time post infection, illustrated as a solid line for the "historical" strains. It is typically at its highest around 5 days post infection. Variants alter this profile and may confer a higher level of transmission (dashed line) or earlier transmission (dotted line). © François Blanquart.
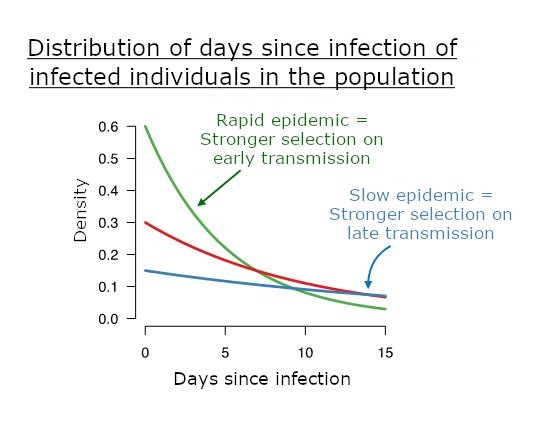
The bottom graph shows time distributions post infection among infected individuals in the population for a fast or slow epidemic. A fast epidemic leads to a majority of recently infected individuals and higher selection for early transmission. © François Blanquart.
This study is part of the priority scientific area Emerging infectious diseases of the Institut Pasteur's strategic plan for 2019-2023.
Source :
Selection for infectivity profiles in slow and fast epidemics, and the rise of SARS-CoV-2 variants, eLife, May 19, 2022
François Blanquart1, Nathanaël Hozé2, Benjamin John Cowling3,4, Florence Débarre5, Simon Cauchemez2
1 - Centre for Interdisciplinary Research in Biology (CIRB), Collège de France, CNRS INSERM, PSL Research University, Paris, France
2 - Mathematical Modelling of Infectious Diseases Unit, Institut Pasteur, Université de Paris, UMR2000, CNRS, Paris, France
3 - WHO Collaborating Centre for Infectious Disease Epidemiology and Control, School of Public Health, Li Ka Shing Faculty of Medicine, The University of Hong Kong Pokfulam, Hong Kong Special Administrative Region, Hong Kong, China
4 - Laboratory of Data Discovery for Health, Hong Kong Science and Technology Park New Territories, Hong Kong Special Administrative Region, Hong Kong, China
5 - Institute of Ecology and Environmental Sciences of Paris (iEES-Paris, UMR 7618) CNRS, Sorbonne Université, UPEC, IRD, INRAE, Paris, France